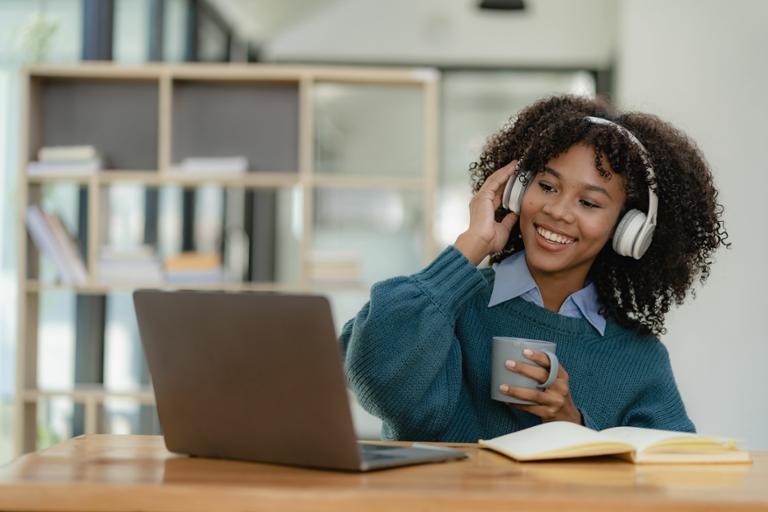
Whatever your feelings about artificial intelligence (AI), one thing is clear: businesses are rapidly adjusting to a reality where AI has been baked into a plethora of workflows. How that might impact your own job is an open question.
A new study by Ernst & Young LLP suggests that A.I. adoption will force 50 percent of businesses to unleash layoffs alongside new hiring in an attempt to adjust. Companies are reshaping their workforce to be more AI savvy. With this transition, we can anticipate a continuous cycle of strategic workforce realignment, characterized by simultaneous layoffs and hiring, and not necessarily in equal volumes,” Vamsi Duvvuri, EY Technology, Media and Telecommunications AI Leader, wrote in a statement accompanying the study’s data. “But it’s not all doom and gloom. Employees and companies alike continue to show enthusiasm around AI, specifically when it comes to opportunities to scale and compete more effectively in the marketplace.”
Of the 255 business leaders surveyed for the study, some 72 percent said their employees were using AI at least daily in the workplace. Top uses included coding and software development (51 percent), data analysis (51 percent), and internal and external communication (47 percent). Moreover, 82 percent of these leaders plan increasing their AI investment in the next year.
Amidst this changing landscape, these leaders are hiring for key roles, including:
Cybersecurity Analyst
AI will increasingly automate threat detection and other aspects of the cybersecurity business, freeing analysts to focus on strategic response and investigation.
- AI Tools to Know: Anomaly detection systems, SIEM (Security Information and Event Management), Machine learning for threat hunting.
- Languages: Python (Scikit-learn for ML), scripting (bash, PowerShell) for automation.
- Takeaway: Upskill in AI to understand and leverage automated tools. Hone soft skills like communication and critical thinking for effective incident response.
Data Scientist
AI will impact data cleaning and feature engineering, allowing data scientists to focus on model building and sharing their results with other stakeholders throughout their organization.
- AI Tools to Know: Natural Language Processing (NLP) for text analysis, Deep Learning for complex pattern recognition.
- Languages: Python (TensorFlow, PyTorch for deep learning), R for statistical analysis.
- Takeaway: Learn AI fundamentals to build and interpret complex models. Strengthen communication skills to translate insights into actionable business strategies.
AI Engineer
Although a relatively new role, AI engineering is evolving quickly. The focus is shifting from designing algorithms to building robust and secure AI systems for real-world applications.
- AI Tools to Know: Deep Learning frameworks (TensorFlow, PyTorch), Explainable AI (XAI) for model transparency.
- Languages: Python (core language for AI development), C++ for high-performance computing.
- Takeaway: Deepen your understanding of AI theory and practical application. Master software engineering principles to build scalable and secure AI systems.
If you want to expand your career to incorporate A.I., consider the following:
- Online Courses: Numerous online platforms offer AI and ML courses with varying depths. Choose reputable providers with career-oriented curriculums.
- Personal Projects: Experiment with open-source AI libraries and datasets to gain hands-on experience. Build a portfolio showcasing your skills.
- Certifications: Industry-recognized AI certifications can validate your expertise and enhance your resume.