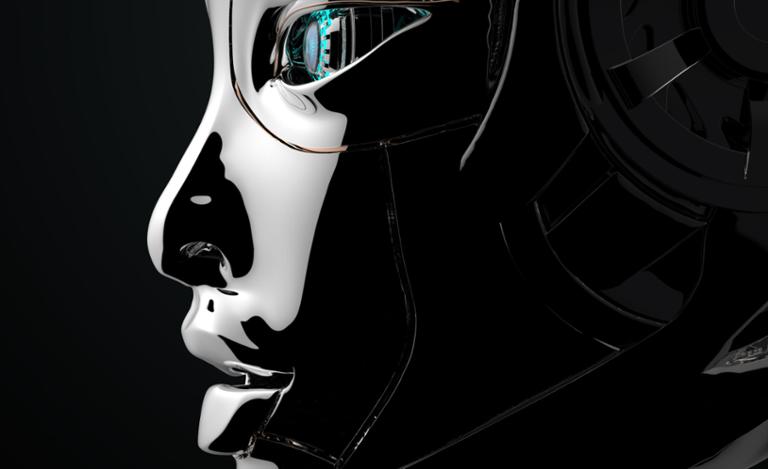
Like many tech companies, Amazon has experimented with machine learning (ML) techniques to improve its recruiting process. But according to a new Reuters report, the company hit a huge snag a few years ago: Its algorithms began preferring male applicants. Reuters based its report on five anonymous sources, who said that Amazon’s ML-based tool rated candidates on a scale of one to five stars. The tool’s algorithms analyzed 10 years’ worth of résumés and assumed that, because the majority of applicants were male, males were preferable hires. As a result, it began downgrading any mention of “women” or “women’s” in résumés. “Amazon edited the programs to make them neutral to these particular terms,” Reuters added. “But that was no guarantee that the machines would not devise other ways of sorting candidates that could prove discriminatory, the people said.” Eventually, Amazon decided to dissolve the team behind the project. If you’re involved in tech, you know that machine learning is having a bit of a moment. Hiring for machine-learning positions is on the rise in major tech hubs such as Silicon Valley and New York City, and machine-learning experts are pulling down very hefty salaries (comfortably in the six figures per year, in many cases). That sort of heady activity can lead even the most cynical technologists to believe that machine learning models can solve even the most intractable problems. But ML and A.I. are yet another case of “garbage in, garbage out,” especially if the core datasets are noisy. That places a market premium on data scientists and data analysts who know how to wrangle massive amounts of data, and study the output from ML models to ensure it’s what the company actually wants. For companies and tech pros alike, there’s a huge lesson in Amazon’s experience. Machine-learning platforms are shiny and cool, but they’re as fallible as anything else. Even the most learned tech pros should take some caution when evaluating models and outputs.