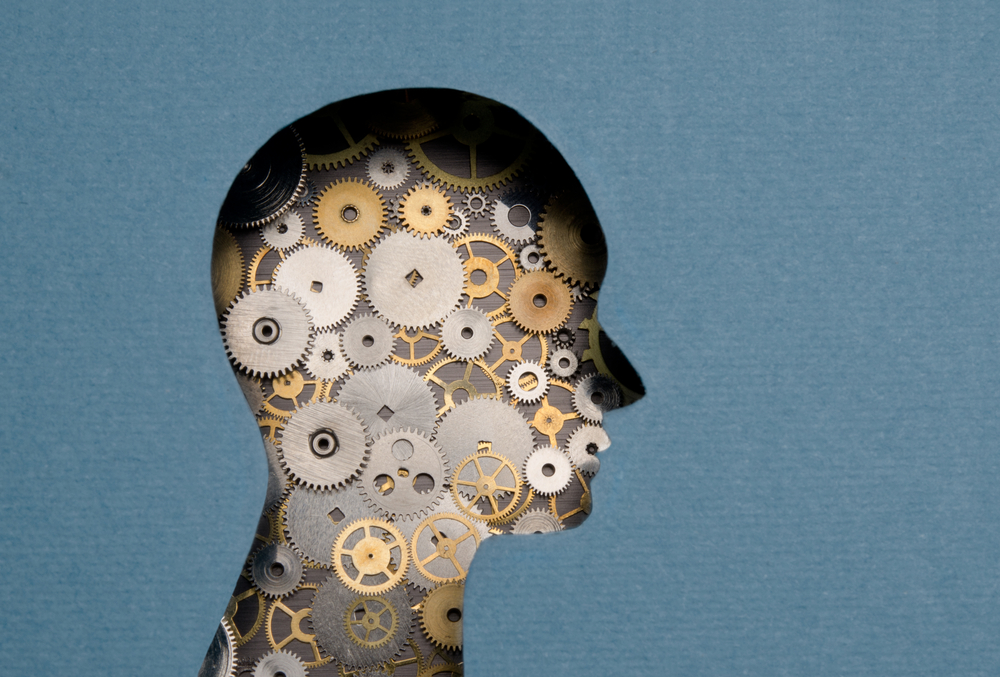
According to a
Siemens study, the global market for “smart” devices is expected to increase 20 percent annually over the next eight years. The boom is driving a need for tech pros with artificial intelligence (A.I.) and machine learning experience in both startup and established-company spaces. While A.I. and machine learning can be fairly broad terms when applied in a general job description, it’s fair to say that data scientists and software engineers are especially suited for finding a role in this arena. Needs and expectations may vary by organization, but a comprehensive understanding of data analytics and tools is a common denominator. Tim Porter, founder and CEO of Gluru, an analytical and predictive data software company whose Web and Android platform enables users to call up or organize essential files, calls and meetings in real time, suggested that technology pros interested in this segment should learn the fundamentals of software engineering, in addition to machine learning and natural language processing. Porter has noticed an influx of database administrators into the machine-learning field. They adapt quickly, he’s observed, because they understand the sheer magnitude of the libraries that have to be accessed accurately and quickly for the software to work. Data scientists with industrial or academic research experience are sought-after at Jetlore, a global provider of personalized content orchestration for relationship commerce. Eldar Sadikov, the company’s CEO and co-founder, stresses Jetlore’s highly technical DNA. “Our needs may be different from let’s say an established retailer,” he said. “We first want someone who’s done research in a data intensive environment, where they had to work large, large data sets and solve problems or build models that capture insights gathered from research, for problems that are not toolbox things.” Sadikov also wants tech pros with strong engineering skills. “The nature of industry right now is that there are a lot of smart people with quantitative skills,” he said, “but you also need to complement them with engineering skills. It's not that you just run all of the experiments in MatLab and that's all you've got.” Jetlore wants to see how tech pros have implemented ideas on real datasets, and used real programming languages and frameworks. Software skills are dependent upon the role, and every company has different needs. “It depends on which way the wind is blowing,” Porter suggested, “but the current popular languages are Python, Java and Go for machine learning and open source tools such as TensorFlow are great.” “I like to see C++, Java, Scala,” Sadikov added, while emphasizing his company’s heavy use of Spark, which allows it to analyze computational infrastructure across servers and the cloud. Porter and Sadikov want their teams to iterate on innovation rapidly, so both consider agility a critical soft skill. Many good data scientists are used to working on a problem for 6-12 months; but the rapidly evolving nature of the industry means shorter timeframes for progress. That’s not to say that some problems take longer to solve than others; patience is another indispensable characteristic, as is being able to clearly articulate problems and insights.
Workflow
Because Gluru frequently delivers new features, often involving integrations or expansions to new platforms, it evaluates individual team members’ strengths before assigning them to a particular task. There’s also a great deal of peer review; taking an OKR (Objectives and Key Results) approach allows the company to evaluate delivery in a qualitative and quantitative way. Jetlore uses similar tactics. “We have director of data science and then we have data scientists within the team,” said Sadikov, who runs weekly meetings. “I give suggestions and the team gives their own input and we discuss and make a plan and iterate from there.” They also take advantage of engineer and data scrums; the data science team will listen in on engineering meetings so there's mutual understanding at every level of a project. You know those emails you get from Ebay that uncannily predict your wants and needs? That’s a Jetlore production, and it’s illustrative of what happens when best practices in predictive analytics and machine learning collide. This project is particularly interesting because Jetlore optimized a model that works across 80 or 90 different countries, encompassing different languages and shipping rules while remaining personalized and accurate. “The users remain highly engaged and they click more through those emails and ultimately buy more because of us,” Sadikov said. “We're very proud of the work that we've done with them.” And none of it would have been possible without deep knowledge of machine learning and A.I.