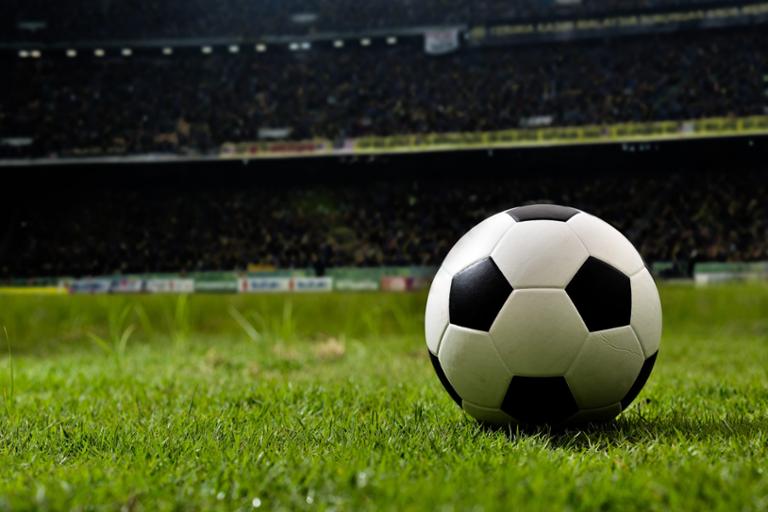
If you’re a fan of the World Cup, you probably had your sights set on a winner before the tournament kicked off. Maybe you really liked how Spain’s team was shaping up (despite the coaching shifts), or you wanted to root for an underdog such as Japan or Croatia. Goldman Sachs, which knows a little something about probability and risk, built a sophisticated data model to predict the World Cup’s eventual winner. This model leveraged machine learning to simulate 1 million possible evolutions, and updated throughout the tournament, according to Bloomberg. With that kind of setup, you’d think that the algorithms would get at least a few match outcomes right. But the Goldman Sachs model, despite its massive dataset, despite the data experts working on it, got its predictions wrong. And therein lies the issue: Although pundits and experts have made bold predictions about how artificial intelligence (A.I.) and machine learning will change our lives in the years and decades to come, these technologies remain relatively nascent, and often unproven in terms of results. It’s not just a matter of predictive analytics such as soccer scores; practical applications of A.I. such as autonomous driving have hit their own roadblocks (pun intended). In March, for example, one of Uber’s autonomous vehicles crashed into a pedestrian, killing her; Tesla vehicles have likewise collided with obstacles while driving in “autopilot” mode. No wonder some 73 percent of Americans said they were “too afraid” to ride in a car entirely under software control, according to an AAA survey. If enough companies (and potential consumers) begin to think that A.I. is overhyped, they’ll spend less on the technology, leading to an A.I. “winter.” The tech industry has gone through such periods before, notably in the 1970s and ‘80s; agencies and universities would pull funding from promising projects, draining energy from the field. At the moment, A.I. and machine learning is making a bit too much money to “freeze up” quickly—look no further than Amazon Alexa and Google Home, which are spreading into millions of households. Within corporations, more and more enterprise applications are incorporating predictive analytics and other facets of machine learning. But as the Goldman Sachs soccer model demonstrates, these technologies still have quite some distance to cover in order to meet the rosiest predictions—and some companies may well give up on their efforts. But if Goldman Sachs sticks with it, maybe their algorithms will get the World Cup right in... 2026.