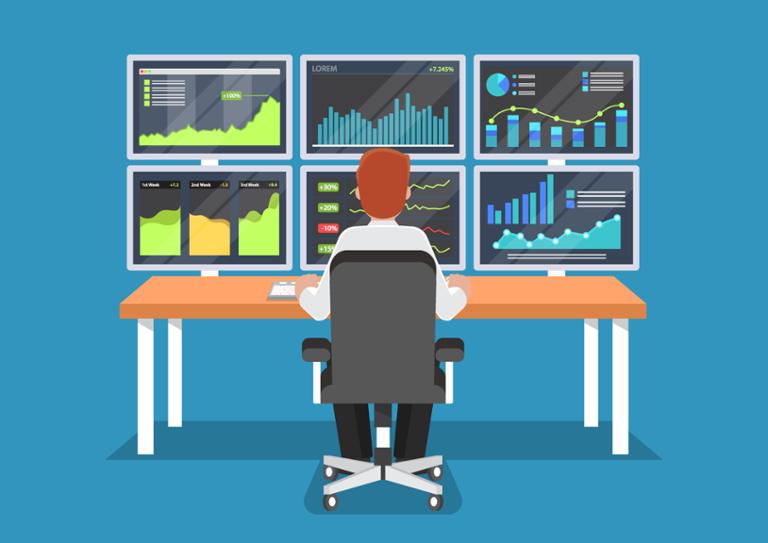
This article excerpt is from eFinancialCareers. If you want to work on J.P. Morgan’s Quantitative and Derivative Strategy team within the bank’s Global Research group, then you better have a PhD in one of three areas: applied mathematics, computer science, or statistics. That’s according to Rajesh Krishnamachari, a vice president of quantitative and derivatives strategy—a quant investment strategist and researcher using machine learning and Big Data-based investing—at J.P. Morgan Chase. He gave an afternoon keynote address at The Trading Show New York. “Our team has PhDs in different disciplines—there are a couple with PhDs in applied math, who work on computational geometric algorithms, PhDs [in computer science] with the deep-learning skills we’re trying to enhance on, and PhDs in statistics, who apply classical mathematical techniques to writing algorithms,” Krishnamachari said. “[That said,] do not discount the value of fundamental knowledge, because the fundamental narrative does not change—we’re still looking at data inputs such as GDP, what central banks are doing and geopolitics. “When a PhD joins the team, I assume that I’m going to train them on economics and finance,” he added. “Rigorous mathematics might not lead you to the right answer, so even when you hire people with the backgrounds I mentioned, you have to make sure they have the right markets intuition.” Krishnamachari said that he and his team work on classifying nascent datasets—assessing the relevance and applications of various datasets generated by individuals, businesses and machines; and advanced machine-learning algorithms—supervised learning, unsupervised learning, deep learning and reinforcement learning, and processing alternative data. “Data can come from individual people, machines or groups of human beings who have organized themselves into business processes,” Krishnamachari said. “To understand the world, we have to classify data via a taxonomy, for example, classifying data by the asset class that it’s relevant to: equities, commodities, credit, rates or FX. “Some data might night be viable as a stand-alone signal but they would be in the context of a portfolio.” A must for success in this role? You have to know your algorithms and the provenance of the tools employed in modern financial data analysis, including statistics, electrical engineering, computer science and financial econometrics. “Machine learning is just advanced statistics from a computational perspective,” Krishnamachari said. “Classical tools are still valuable in the age of machine learning and artificial intelligence.” For more on the lessons that Krishnamachari teaches his team, including the relative complexity of algorithms, check out the article on eFinancialCareers. It was originally written by Dan Butcher.