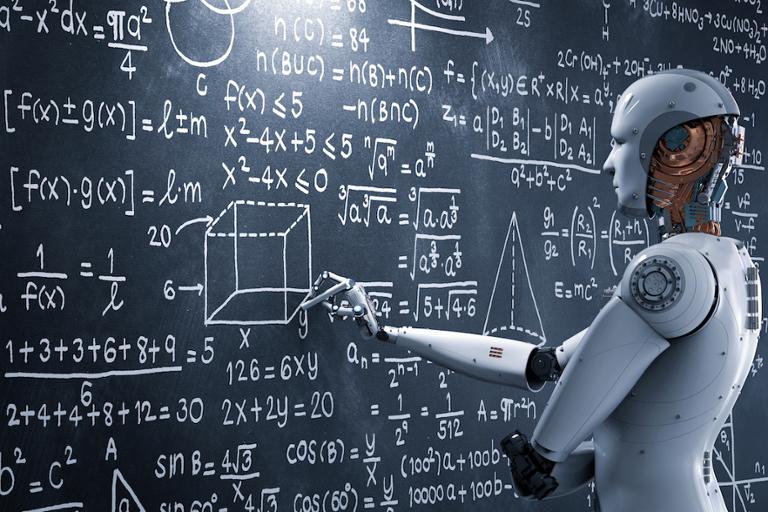
Will artificial intelligence (A.I.) and machine learning carve up the tech industry into “haves” and “have nots”?
That’s the thesis presented by a recent article in The New York Times, which suggests that, while ultra-monetized companies such as Google and Facebook can fund as much A.I. research as they need, academic institutions and smaller firms are being left behind. “The huge computing resources these companies have pose a threat—the universities cannot compete,” Craig Knoblock, executive director of the Information Sciences Institute at the University of Southern California, told the newspaper.
The Times points to OpenAI, which launched as a nonprofit designed to prevent A.I. from being used in terrible and unethical ways, as an example of this trend. OpenAI has since evolved into a “capped” for-profit company, and reportedly plans to use any revenues to fund its computing infrastructure. “If you don’t have enough compute, you can’t make a breakthrough,” Ilya Sutskever, chief scientist of OpenAI, is quoted as saying.
It’s worth noting that Sutskever made nearly $2 million in 2016, according to reports, while another OpenAI researcher pulled down $800,000. In fact, it’s worth examining salaries and benefits as a crucial element in this particular mix—something the Times article doesn’t really delve into. The ability of big companies to offer millions of dollars in salary and stock compensation to individual A.I. researchers is a huge advantage, one that’s almost impossible for smaller companies—and all but the richest research institutions—to meet.
Compounding the issue are the working conditions. As one anonymous A.I. researcher wrote on Hacker News:
“So I am a machine learning researcher who moved to a FAANG as a research scientist after graduation. My salary is 10x against the grad student stipend. That does not even account for the free food, the healthcare, and other perks. However, I have not adjusted my lifestyle so it does not feel real.
The thing is, even though having 1000x the resources compared to university, that does not really make me happier about the work specifically. It makes some things easier and other things harder.
No, what I really feel is that at work I am not actually treated like a servant any more but like a person. I don't have to work weekends and nights any more. I can take vacations and won't be flooded with emails every.single.day during holidays. I don't have extra unpaid responsibilities that I have zero recourse against.”
For a technologist well-versed in everything A.I.-related, trying to decide whether to work for an academic institution with grinding hours and relatively little compensation, or a fancy company with lots of money and perks… well, it’s probably a pretty easy decision, especially if they’re also facing down a huge load of student debt.
At least academic institutions have a slight advantage here. While an A.I. research team might not have millions to spend on infrastructure and salaries, they often have access to a well of brilliant researchers and up-and-coming talent. As A.I. and machine learning become more popular, more smart people will head to universities to learn the intricacies of these technologies; their brainpower, in turn, can help academia at least somewhat keep pace with the likes of Google and Facebook.
Smaller companies, however, are at much more of a loss. A startup with ten employees and a brilliant idea for an A.I.-powered service might find it impossible to pay for the necessary hardware and researchers. (For example, the just-released IEEE-USA Salary & Benefits Salary says that engineers with machine-learning knowledge are making an average of $185,000 per year—and that’s before you consider equity, bonuses, and other perks. Multiply that by 15 or 20, and there’s no way a small company can make that kind of payroll.)
The biggest tech companies recognize this challenge facing startups and small firms, which is why many are rushing to develop tools that allow workers to build A.I. and machine-learning models with just a few clicks. For example, Google is plunging into the ML-automation game with AutoML Video and AutoML Tables; Microsoft has automation and recommendation tools built into its Azure Machine Learning platform; then there’s IBM’s AutoAI, with a handful of tools for building A.I. and machine learning algorithms.
But while such tools might allow those smaller firms to build “smarter” platforms and tools, they might be out of luck when it comes to pioneering research. When it comes to the absolute cutting edge of technology, only a few companies have the necessary resources to plunge ahead. But hasn’t that always been the case?