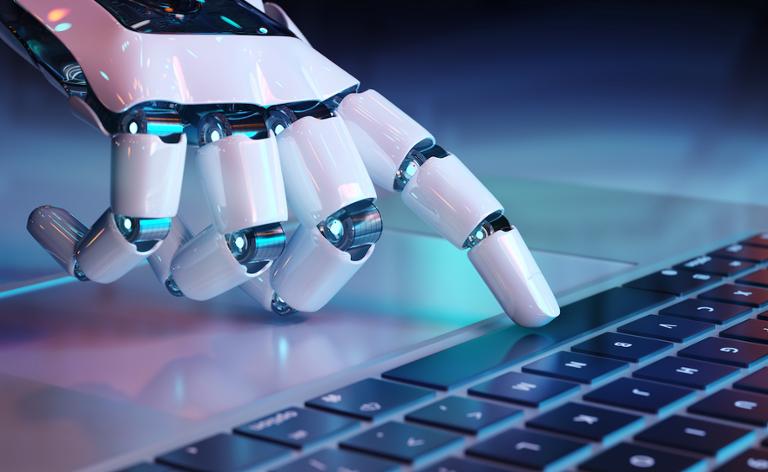
Artificial intelligence and machine learning are reshaping industries, creating new opportunities, and driving innovation. For tech professionals everywhere, the impact of this technology is already clear: for example, coders are already using generative AI tools to help them produce code faster than ever before.
At the same time, AI still seems relatively nascent as a tech segment. With every passing quarter, many of the AI tools on the market get more powerful, but they can’t quite deliver on their creators’ world-changing promises (not yet, at least). With that in mind, is it the right moment for tech professionals to start learning AI and machine learning?
Summary
Why You Should Get Into A.I. and Machine Learning
Soaring Demand, Lucrative Rewards
Job Market Boom: Roles in AI and ML are in high demand. Data scientists, AI engineers, and ML researchers are sought-after by top tech companies and beyond.
- Salary Surge: AI/ML professionals often command significantly higher salaries than their peers in traditional tech roles. From prompt engineering to model creation, AI skills are powering significant rises in compensation for those who take the time to learn them.
Industries Revolutionized by AI/ML
Healthcare: AI has the potential to revolutionize diagnostics, drug discovery, and personalized treatment plans.
- Finance: AI tools are already helping tech pros in finance discover fraud, optimize trading strategies, and predict market trends. While some version of machine learning has been used in finance for more than twenty years, recent tech advances have supercharged AI use in this industry.
- Retail: From enhancing customer experiences to optimizing supply chains and personalizing recommendations, AI will impact retail in a big way. For example, tech pros with AI skills have been tasked with making customer chatbots “smarter” through the use of deep learning and other AI tools.
- Autonomous Vehicles: The development of self-driving cars and other autonomous systems has been going on for quite some time, but big steps in data curation and analysis is allowing companies to move further, faster.
How to Start Your AI/ML Journey
Building a strong AI knowledge foundation is critical. It also depends on your ultimate intentions with AI. For example, those who just want to use the current crop of AI tools effectively can just focus on prompt engineering. However, those who actually want to build and iterate AI systems need to master some combination of the following skills:
- Programming Proficiency: Master Python, the go-to language for AI/ML.
- Mathematics Mastery: Grasp linear algebra, calculus, and probability theory.
- Data Science Fundamentals: Learn data cleaning, exploration, and visualization.
Choose Your Learning Path
Online Courses: Platforms like Coursera, edX, and Udemy offer a wide range of AI/ML courses. Best of all, many of these courses come at a reasonable cost and feature lots of help.
- Self-Guided Learning: Utilize free resources like YouTube tutorials, online documentation, and open-source projects.
Dive into Practical Projects
Build a Portfolio: Create projects that showcase your skills, such as:
- Image classification with convolutional neural networks
- Natural language processing with recurrent neural networks
- Recommendation systems with collaborative filtering
- Time series forecasting with ARIMA or LSTM models
- Participate in Kaggle Competitions: Test your skills against others and learn from top-notch data scientists.
Stay Updated and Network
Follow AI/ML Blogs and News: Stay informed about the latest trends and breakthroughs. Newsletters such as AI Breakfast, Mindstream, and The Rundown AI are all good places to start.
- Join Online Communities: Connect with other learners, share knowledge, and seek help. For example, subreddits like r/AIAssisted and r/ChatGPT are good places to start.
- Attend Conferences and Meetups: Network with industry professionals and expand your knowledge.
Overcoming Common Challenges
As you might expect given its complexities, the AI arena presents a number of challenges. As you learn more about how the technology works, these might slow down your learning—if you let them.
- Complexity: AI is massively, intimidatingly complex. As with learning other kinds of technology, the key is to break down complex concepts into smaller, manageable parts.
- Technical Prerequisites: Yes, many aspects of AI require quite a bit of specialized knowledge. It’s critical to start with foundational courses and gradually build your skills.
- Lack of Practical Experience: Work on personal projects and participate in hackathons if you want to boost your hands-on work with AI.
Conclusion
With dedication and consistent learning, you can unlock a world of opportunities in AI/ML. Whether you aspire to become a data scientist, machine learning engineer, or AI researcher, the future is bright for those who embrace this transformative technology.