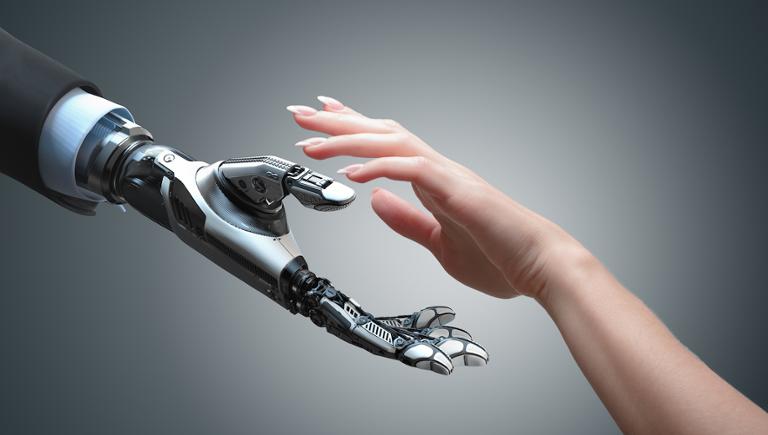
On June 8, 2022, Accenture presented The Art of A.I. Maturity report. The report revealed that only 12 percent of companies surveyed use A.I. at maturity level, achieving superior growth and business transformation. 63 percent of companies using A.I. are only scratching the surface.
While A.I. can provide significant benefits for Enterprise organizations across any sector, the potential of the technology is still far from reaching its peak. While multiple problems can trip up your Enterprise AI adoption, there are four key challenges that companies will face as they move into 2023. Understanding these challenges can help organizations build a road map and their A.I. strategies. They are the difference between mastering A.I. and reaping the benefits or merely playing with a tech novelty.
Creating a Business-Driven A.I. Culture
Companies excelling in machine learning and solving real business problems with A.I. will likely have a strong innovation culture across all levels. Currently, hundreds of thousands of companies are experimenting in some way or another with A.I. Within these companies, teams of data scientists or data engineers are in charge of leading the way forward by developing machine learning models that benefit the company. However, these teams are often isolated, develop models in a very ad-hoc, almost artisanal fashion and are disconnected from decision-makers, compartmentalized, and have little support from C-suite executives or other departments.
Companies that start A.I. projects as experimental and later pitch them to their organization have higher failure rates than those with initial approval for production. Data teams should get input from top decision-makers on the challenges the company faces and build machine learning models that address these real-world business problems.
Data scientists working in organizations that do not have a strong A.I. culture often conflict with the “old ways of doing things.” Blackbox A.I. projects may not get buy-in from executives because they fail to understand how the machine learning model arrived at its results.
Skilling all workers, from CEOs to IT, marketing, sales, and office workers, breaks the language and technical barrier and creates support and understanding. Data scientists within an organization can not work alone. They need to collaborate with other departments.
Moving from Experimental AI Projects to Production
There are a myriad of reasons why organizations struggle to move A.I. experimental projects into production. Most business challenges are directly linked to the lack of a strong A.I. culture. This generates all kinds of problems.
For example, CEOs often do not understand the limitations of A.I. and what it can and can't do. For example, machine learning models can impact a company’s bottom line — especially in the current economic climate and the looming recession — through A.I. solutions that optimize marketing spending through marketing mix modeling or models that can lower customer churn. But other prediction problems may not be solvable for several reasons, like insufficient data.
It is also vital for management to understand how machine learning models work and how much time it takes to build a model. Building machine learning is time-consuming. The “2020 State of Enterprise ML” report of Algorithmia revealed that only 63 percent of 745 companies surveyed deployed machine learning models into production. On average, 40 percent of companies said it takes more than a month to deploy a machine learning model into production.
Data scientists must go through vast amounts of raw data, choose what data they should use, speak to experts, train their models, and benchmark results. Suppose executives are looking to solve a business problem with a time-sensitive deadline, for example, predicting recession impacts in the next three months. In that case, they must consider the time limitations of developing A.I. models. These time-consuming processes can lead to frustration and often make A.I. experimental projects hard to follow through.
On the technical side, experimental projects often fail to go into production because they use a subset of much bigger data. An experimental model may work well with a subset of data. Still, other variables come into play when it goes operational and uses live data, and the model may drift, fail, or collapse. Another issue with operationalizing models is that data science code is initially in Python — a high-level, interpreted, general-purpose programming language. Converting machine learning code into something that can be used in production systems can be time-consuming and difficult.
Investing in Talent, Technology, and Data Environments
As A.I. and machine learning have become mainstream, new companies that offer A.I. services began to rise. Machine Learning Automation platforms are trending for their potential to accelerate and augment the work data scientists do.
Four key benefits of new A.I. solutions worth focusing on are: discovery of patterns, building data pipelines, validating features, and business insight discovery. New technologies that empower discovery of patterns in data (features) can give data engineering and data science teams the ability to quickly move from raw, relational enterprise data to the flat tables machine learning needs. Building data pipelines for ML relates to the ability to feed feature stores with new and up-to-date Machine Learning-ready features that can be used across as many use cases as possible to accelerate development time.
Data scientists can also reduce development time and provide better models with already vetted features by validating features for machine learning to identify how "valuable" a feature might be for any given model. Finally, new solutions can drive the discovery of business insights.Machine learning crunches massive amounts of data, but while not all may be valuable, these new technologies can find patterns in data and use them even outside of the realm of machine learning.
While new A.I. technology can accelerate and improve processes, A.I. also presents challenges for companies. One of these is transparency. A company may develop a complex model to predict sales and demand or manage inventory but may not understand how the model works. This is a problem for many reasons. If we can’t understand how models work, how can we trust the results or recommendations it is providing?
The other problem with automatically discovered features is that companies may wrongly believe these solutions can replace their talented teams and investments in ML technology. A.I. automation platforms are tools developed for data scientists, not their replacements. With these tools, data scientists can become more productive and eliminate many of the frustrations that slow development processes, doing in hours what traditionally has taken months to complete..
Responsible AI and Transparency
The concept “Responsible A.I.” has become a buzzword for the industry, with companies like Google promoting slogans like A.I. that “does no harm” and Microsoft referring to its cloud as a “cloud for global good.” However, there is more to these slogans than what meets the eye.
From the start of the project, leading A.I. companies think about how they will use their machine learning models and what data they will use. There are several ways in which a machine model can harm. It can underperform and negatively impact an organization or be coded without an understanding of unintended consequences of its use.
For example, a credit scoring company developing a machine learning model to give clients a credit evaluation in just seconds must consider what data is ethical to use. While gender, race, zip code, or other data could impact a credit score that would influence whether a client should get credit, data scientists must ask themselves if using such data is ethical.
Machine learning models and A.I. do not have inherent concepts like “responsibility” or “ethics” and can discriminate and be biased. There are ethical responsibilities that come with developing and using machine learning models. Transparency is critical in ensuring ethical use of ML models. It is vital for everyone inside the company, not just the data scientists, to understand how an algorithm is arriving at its conclusions and what data was used in the process.
When a machine learning model can solve highly complex problems, but humans have challenges understanding how a model reaches conclusions or makes predictions and what data it uses, it lacks transparency. These so-called “black box” A.I. models can be problematic. A.I. development platforms must provide feature transparency and traceability. The first refers to the ability of users who did not develop the model to understand how it arrived at its predictions. Traceability, on the other hand, refers to the ability of developers to “trace” the data elements that were used in specific predictions and in specific features associated with any given model.
Machine learning must be easy to understand, transparent, responsible, and accessible. The four interconnected challenges that the industry, and the world, face today will shape our future.
Creating a business-driven A.I. culture, efficiency moving from experimental A.I. projects to production, investing in talent, technology, and data environments and embracing the concept of responsible A.I. from the start is how organizations are mastering machine learning.
Ryohei Fujimaki is Founder and CEO of dotData.