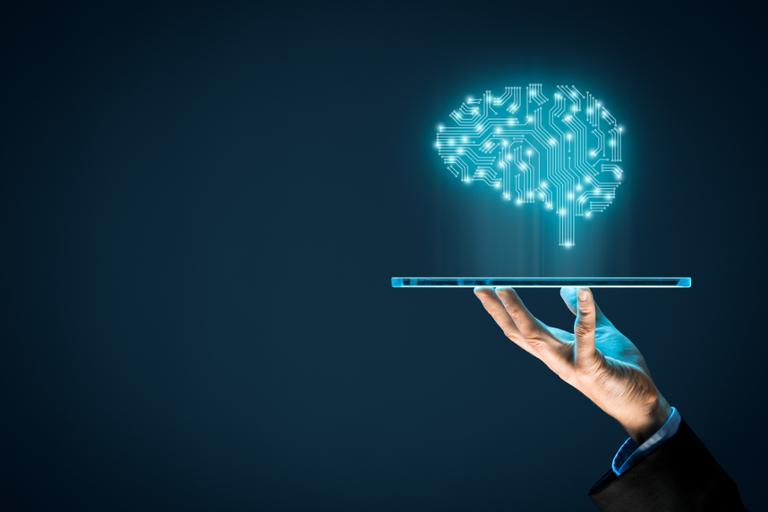
Imagine there’s a pot of gold in the middle of a room. In one corner is the Easter Bunny. Santa’s in the second corner, an unbiased artificial intelligence (A.I.) platform in the third, and you’re in the fourth. Who gets the gold?
You do. Because there’s no such thing as the Easter bunny, Santa Claus or an unbiased A.I.
Maybe it’s a tacky joke, but it nonetheless spotlights a little-discussed aspect of artificial intelligence. While data scientists often talk about how important clean data is to the effectiveness of A.I. systems, they’ll also say that even the most advanced A.I. has biases programmed into it. That’s not because developers are purposely skewing their software’s logic. It’s because developers, like all other people, bring their own preconceptions to the table; consciously or unconsciously, their work is going to reflect those presumptions.
“We don’t know how to assess bias and there may not be any systems or people out there who are truly bias-free, so it’s unreasonable to expect a programmer to produce code that somehow achieves the holy grail we can’t produce ourselves,” said John Harney, CTO of New York-based DataScava, developer of an unstructured data miner.
That’s an important thing to recognize when so many experts see A.I. as a promising way to eliminate or mitigate bias in everything from recruiting and hiring to urban planning. A growing number of data scientists believe that, in reality, A.I. can’t be left to its own devices. Instead, they say, the systems must be paired with users who’ve been educated about its potential pitfalls. While the technology itself should have a high-level “sense”of how an organization defines bias, it’s important to remember that A.I. doesn’t take human decision-making out of the loop. As one expert said: “It doesn’t abdicate human responsibility.”
Building Bias Awareness (in Humans)
In addressing the situation, awareness plays a large role, said Dennis Mortensen, founder and CEO of X.AI, a New York solutions provider that applies A.I. to time-management applications.
For developers, recognizing that datasets include biases provides an opportunity to identify the most dramatic issues and determine “how we can, if not eliminate them, at least make them less impactful,” Mortensen said.
It’s also important to define the system’s objectives as clearly as possible. For example, a bank may have 1,000 employees evaluating credit applications. That bank, Mortensen added, “will end up with a probably 1,000 distinct types of decisions, some of them good but some of them probably very bad.” By studying those employees’ work, the bank can examine each decision point and identify at least some of the associated biases. That, in turn, provides information that can be used to remove some bias from the data.
The operative word here is “some.” Bias, Mortensen explained, embeds itself into multiple data points, not just one. That makes it tricky to identify. For example, a user who doesn’t apply bias based on race may be “naïve enough to believe that geography is independent of race.” That’s why downgrading credit card applications based on the applicant’s neighborhood may end up adding a racial component to the decision when none was intended.
Give Users Context
It’s just as important for end users to understand the dynamics of bias and A.I., data experts say. For instance, recognizing a system’s objectives is as important to end-users as it is to developers: When users understand what AI is meant to accomplish, they can more easily identify some of its pitfalls.
Mortensen uses this example: A video-streaming service may offer a recommendation engine designed to encourage subscription renewals. To do that, the system must prioritize titles that closely align with the user’s viewing habits. However, the company could water down the application’s effectiveness if it prioritizes programs that earn higher royalties over the user’s preferences. “If you really want to keep me as a customer for as long as possible, you can’t recommend only things I don’t want to see,” Mortensen observed.
When users—such as bank underwriters—appreciate that concept, they begin to view their technology through, in Mortensen’s words, “a different set of glasses.” They can understand the system in a way that’s similar to how they understand a colleague. When co-workers understand where others are coming from, they collaborate more effectively. The reason: They understand what the other is trying to achieve. A similar dynamic is at play with A.I. and data.
Educating users about unconscious bias is trickier. To start, they have to recognize that A.I. systems are “all just pieces of software” and distinguish between those that present a single conclusion versus those that provide suggestions. “I would be very hesitant in blindly following any system,” Mortensen said.
For example, a software system that screens 1,000 job applicants and presents 80 profiles for review should be seen as an assistant, not a decision-maker. Although the A.I. determined those 80 candidates are particularly strong, users still must vet the system’s conclusions, in part by looking through some of the candidates who were passed over. There’s value, Mortensen said, in “getting a feel for what’s been discarded.”
When educating users, a good first step is for developers to emphasize that for all of its power, A.I. is simply software. “Don’t anthropomorphize it,” Mortensen said.