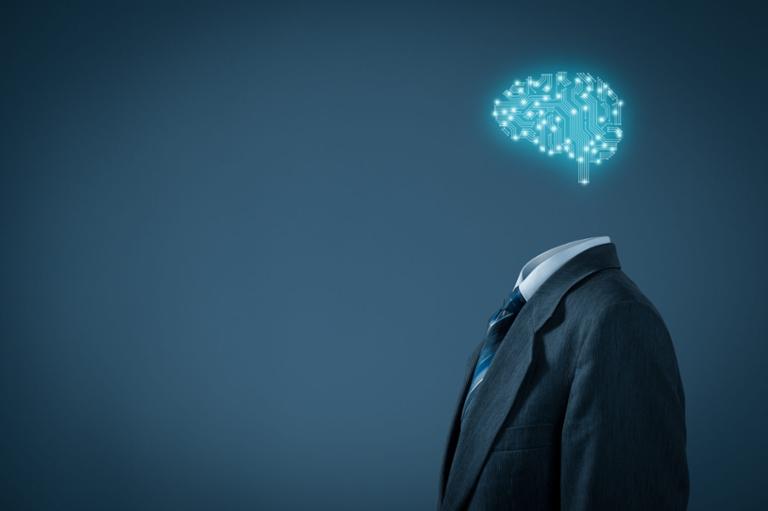
Hiring a diverse team, replete with people with diverging beliefs and from different cultures and of various races, is important because it creates a heterogeneous environment rife with varied perspectives. Indeed, a recent poll of corporate executives found that nearly half of those surveyed are considering compiling metrics around diversity, equity, and inclusion (DEI) in their incentive plans to build a more inclusive and diverse employee base.
But while creating DEI-centric programs is all well and good, the fact is that the focus on DEI must begin well before new employees are hired. Unfortunately, the very beginning of the onboarding process—when a person first submits their resume for consideration—is also a time when bias is all too often present. Studies have shown hiring managers were less likely to call back applicants with gender-neutral or ethnic-sounding names. Meanwhile, other research shined a light on how different words used in resumes from men and women contributed to the hiring gender gap.
Many organizations have incorporated artificial intelligence (A.I.) into the resume-assessment process in the hopes of addressing this propensity for bias. A.I. offers the promise of combing through resumes with a detached eye and singling out candidates based on hard data, such as their background or previous accomplishments. Applicants can be analyzed based on their skills and successes, not their names and genders. Assessments can then be passed along to hiring managers, who can make their decisions based on facts, not their own subconscious prejudices.
That’s the idea, anyway. The reality is that there is still some work to do before A.I. becomes a truly invaluable recruitment tool—starting with ensuring the data that the A.I. is using is bias-free.
Garbage In, Good Candidates Out
An A.I. algorithm is only as good as the data that’s fed into the system. The problem is that the data is being fed by humans and may be somewhat tainted by people’s biases.
For example, most companies use an Applicant Tracking System (ATS) that uses keywords to find ideal candidates for each open position. Those keywords are determined by hiring managers. So, a manager might want to use a term like “aggressive,” “strong,” or “mastery”—words that are typically associated with male applicants. Indeed, a study by Oleo and University College London found that “90 percent of the top 10 words men used in their resumes are proper nouns and common nouns, versus only 68 percent on women’s resumes.”
As a result, in many cases deserving candidates may be shut out before they’re even considered for a position, simply because the keywords they use on their resume do not match what’s in the system. That’s why it’s important for companies to a.) ensure the data being put into the system is free of bias and b.) continue to consider “soft skills” as an integral part of the hiring process.
Mitigating Bias in Data
Filtering out bias in data sets is a significant challenge because people are all, to some extent, naturally biased. There are studies, infographics, and even a theater play attesting to this fact. The question is, how can companies minimize biases to the extent they are not reflected in A.I. data sets?
Soliciting input from different people is a good start. Hiring managers, recruiters, and data scientists must work together to come up with the right keywords, candidate details, and scoring criteria. When they work as a team, their different perspectives can help offset any potential individual biases that might otherwise enter the equation. Then, data scientists can successfully develop algorithms that are not influenced by a hint of partiality.
Factoring in Soft Skills
A.I. is particularly good at evaluating candidates based on so-called “hard skills” it discovers through keywords, phrases, etc. It’s not good at all at detecting “soft skills”—i.e., how well a person works with others. But soft skills are becoming increasingly important for all positions, including technology-related jobs where developers, IT managers, and others are being asked to interact more with senior management and customers.
Thus, companies need to complement the recommendations of their A.I. tools with the instincts and recommendations of the people who will ultimately be hiring their candidates. Ideally, the A.I. will serve as a first line of offense, finding the best possible candidates in a bias-free manner. Those candidates will then be handed off to hiring managers to be interviewed.
While there’s always a chance that bias will factor into the interview stage, it won’t be the fault of the A.I. The candidate will at least have gotten past the initial resume review stage without being snubbed because of their name, gender, or the school they attended. That alone is progress toward a more diverse and inclusive work culture.
Kelly Demaitre is chief human resources officer at Dovel Technologies.