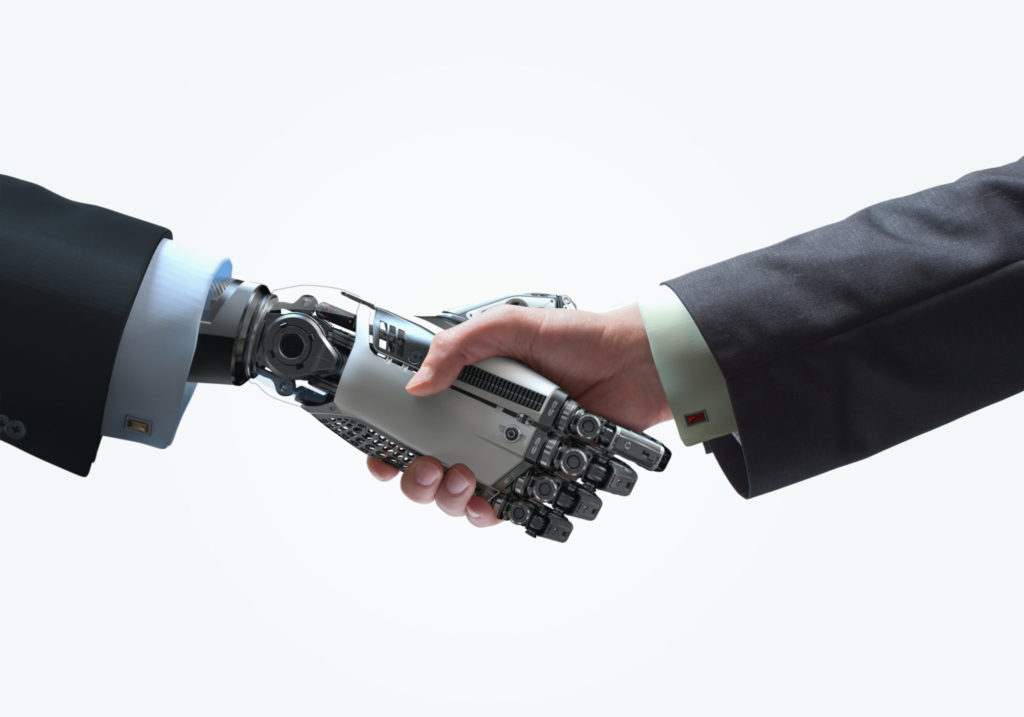
Automation has affected a variety of industries over the past few years, and hiring is no exception. HR departments are beginning to explore artificial intelligence (A.I.) and machine learning as tools for making the perfect hire. The need for this technological edge is especially acute in tech, where firms compete viciously for highly specialized talent, and the right hire can mean the difference between marketplace success and miserable failure. HR has always recognized the need for speed. A long time ago, HR departments began leveraging keyword searches to screen thousands of résumés in a matter of seconds. But even that technique has limits: keyword search only produces hits if the résumés feature the right keywords, opening the door to unscrupulous candidates packing their résumés with terms that don’t accurately reflect their qualifications. In theory, A.I. can “smarten” that search, allowing HR staffers to produce a list of 10 or 20 prospects who really do fit the open position. Understanding how these tools work can help you when refining your application materials for your next job-hunt.
Step One: Start with a Lot of Data
A.I. platforms plow through gigabytes of data in order to arrive at valuable insights. Although these platforms aren’t perfect by any means, data scientists can leverage feedback loops to refine the underlying algorithms. For example, EngageTalent.com and HiringSolved both attempt to leverage A.I. tools to find patterns in voluminous amounts of data. EngageTalent has compiled a dataset of 50 million candidates over the past 10 years, and uses it to trace career paths, job changes and promotions, according to CEO Joseph Hanna. HiringSolved claims it has 480 million profiles that its A.I. uses to train. While all firms involved in A.I. and hiring attempt to collect as much data as possible, key differences emerge in their respective approaches to processing.
Multiple Factors, Multiple Vectors
A.I. platforms—no matter what their target discipline or industry—rely heavily on examining contextual information and linking seemingly disparate elements into a chain of dependencies. When it comes to an A.I. analysis of résumés and application materials, the company that a candidate worked for can prove just as informative as their position or relevant skills. Platforms such as EngageTalent might pull in corporate news in order to add some context to a candidate’s application. For example, a company in distress (such as Uber) will generate job seekers, as qualified tech pros seek a calmer working environment. “Wells Fargo had a lot at one point with the issues they were having,” noted EngageTalent’s Hanna noted. An A.I. model can also identify a person who spent 24 months in the same position and may be getting antsy. That can allow HR staffers to sculpt the language of an introduction email, raising the chances of a response from a candidate. This sort of pattern recognition can apply to all sorts of other aspects of a candidate’s career. A.I. can knock a preliminary hire list of thousands of candidates down to a few dozen—reducing the amount of “human” time necessary to find the best possible candidates.
Machines Do Machine Work
“Everything we do is to look for the balance between man and machine,” said Jeremy Roberts, VP of Customer Experience at HiringSolved, which has an A.I.-fueled HR platform dubbed RAI. “What can a machine do that a human can’t?” Machines are better at sorting through volumes of data, he added. Indeed, any system can match candidates to jobs via a straightforward keyword search. It takes more to formulate accurate recommendations—people a hiring manager would actually want to see—based on large datasets. RAI can examine the problems or challenges that candidates solved in their previous positions, based on their résumés and applications. The hiring manager can then crosscheck their experience against open projects and job openings. “You know the calls are going to the right people first,” Roberts explained. RAI is actively trying to understand the context of the job. With each successive list of qualified candidates, users can refine their searches still further, adding or subtracting qualifications to sharpen the search.
What Candidates Can Do
So what does this mean for job candidates? Fortunately, a well-written résumé, cover letter, and application are always in style—whether those materials are being read by a human or machine. While using keywords (
along with action verbs) is always a good thing, the increasing sophistication of HR software means you don’t have to “lard” up your application with those terms in order to show up on a hiring manager’s radar. In fact, such a move might prove counterproductive if you use excessive keywords to try and land a position you’re not qualified for. Make sure that your résumé is solution-oriented, listing the business problems you’ve solved and the positive results you’ve achieved. Quantifying your victories with numbers and percentages will only strengthen the quality of your profile. Increasingly sophisticated software will pick up on this information, connect it with other data, and (hopefully) shuffle your résumé closer to the top of the stack. And don’t skimp on detail. Although crafting a résumé is often arduous work, listing all the companies you’ve worked for, positions you’ve achieved, and projects you’ve worked on can only benefit you when it comes to dealing with machines.