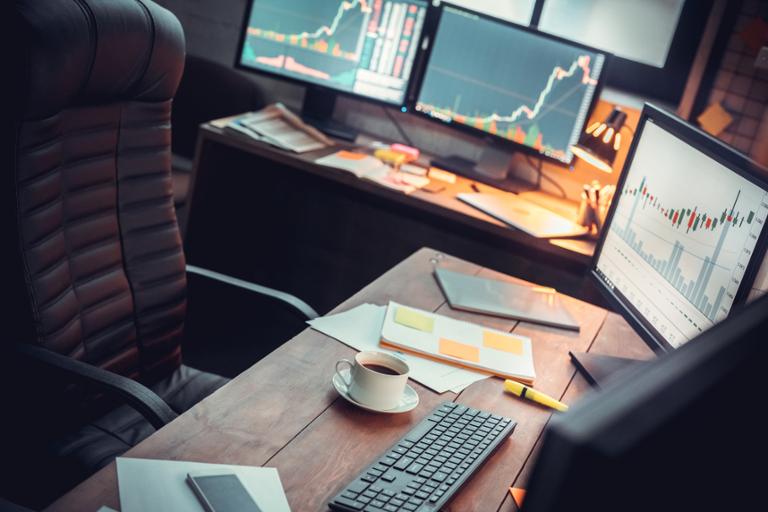
Data science is a rapidly growing area of focus for many companies, and for good reason: The right kind of data, analyzed correctly, can yield insights that translate into better and more profitable strategies. That’s why “data scientist” has become a much sought-after role for companies to fill, with high salaries and generous benefits to match.
Once you begin your data-scientist journey, you very quickly discover that it’s a complicated job, with tons of tools and platforms that could greatly help (or hinder) your data-crunching efforts. The sheer plethora of tools is a little bit intimidating, at least before you build up enough experience to determine what exactly you need in what context.
Fortunately, research firm Gartner has a new, extremely lengthy report that breaks down the positives and negatives of the most prominent data-science and machine-learning tools on the market. While there are some caveats here—for example, the report explicitly excludes tools aimed primarily at application developers and business analysts—it’s a good snapshot of the data-science industry at this particular moment in time.
In evaluating what it calls DSML (data science and machine learning) platforms, Gartner judged how each handled “multiple tasks across the data and analytics pipeline,” including such areas as data ingestion, preparation, and exploration; feature engineering; model testing; and deployment and monitoring.
Along the way, the firm also takes something of a backhanded swipe at artificial intelligence (A.I.), which it suggests is a bit overhyped at the moment. “A.I. hype brings undoubtedly valuable attention and enthusiasm to the data science space. But without education, discipline and reasonable expectations, that hype can do far more harm than good,” the report added. “The vendors… have done a fine job embracing the hype while clearly communicating and delivering value and differentiating their platforms from other A.I. solutions.”
Every platform has its advantages and drawbacks. For example, Google’s Cloud AI Platform offers a number of fantastic tools for data handling, such as BigQuery, as well as machine-learning model creation, including TensorFlow and Cloud AutoML. However, its tools come with a substantial learning curve (according to Gartner) and there’s a lack of project-management support.
In fact, all of these DSML tools, large and small, have a variety of strengths and weaknesses. Tools from smaller firms might provide support for different kinds of technologies and ease-of-use, for example, but struggle with scalability. Platforms from bigger vendors, meanwhile, often do all they can to lock users into a particular tech ecosystem; they also might not adapt well to all potential use-cases.
Where Data Science Meets Machine Learning
If you’ve spent any time in the data science arena, you know that it’s begun to overlap very heavily with machine learning. This shouldn’t come as a surprise; both disciplines involve the wrangling of massive amounts of data, and the automation that results from many ML processes can speed and inform data-science work.
In fact, many data scientists embark on long-term career paths that take them through machine-learning roles on their way to senior positions. For example, a machine-learning engineer could jump to a data science or data architect role (or vice versa) before leaping into senior management. Check out this chart that illustrates this idea:
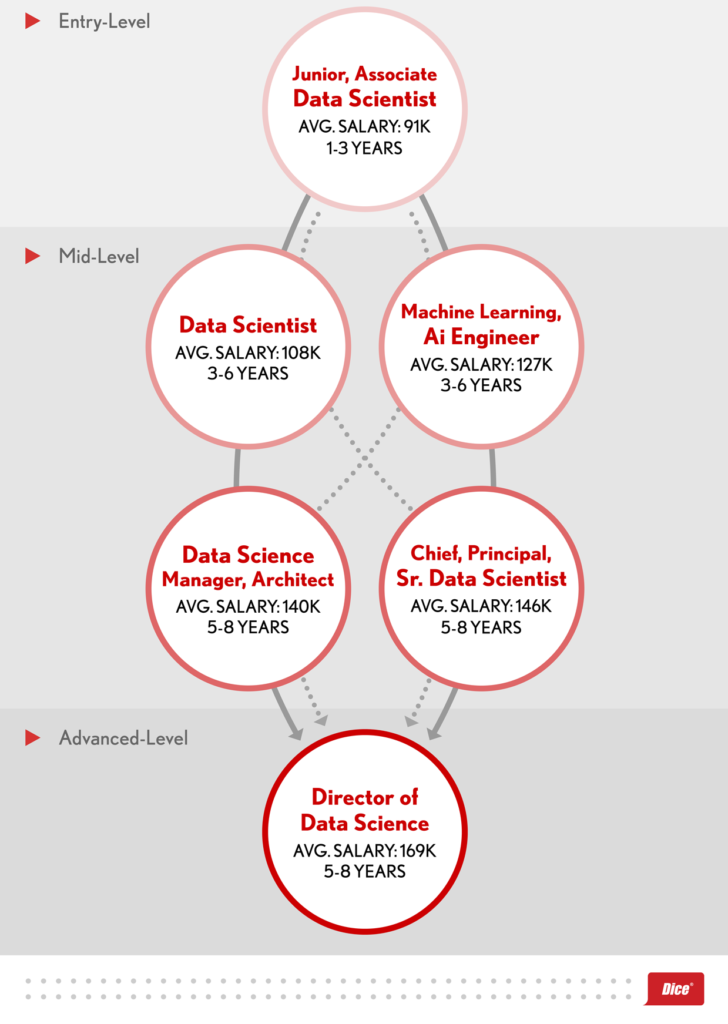
When we recently analyzed Dice’s database, we also found that data scientist salaries and machine-learning specialists closely align, suggesting similar levels of demand (and the aforementioned overlap):
As with so many other disciplines in tech, the key to consistent employment and enviable compensation is specialization and knowledge; those who know their way around certain tools (and DSML platforms) will have a sizable advantage when it comes to not only job-hunting, but also promotions and advancement.