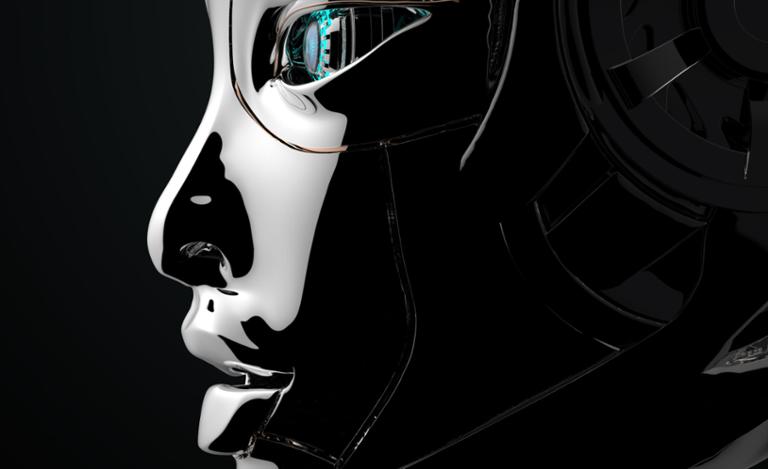
Artificial intelligence (A.I.) and machine learning have evolved from science fiction to something that many tech companies are actively working on. Which means, of course, that the federal government is now looking at whether A.I. needs regulation.
According to Axios, the White House is “warning federal agencies against over-regulating artificial intelligence as part of fresh guidance on how to govern the next-generation technology.” In line with that, the Trump administration is setting out 10 principles for agencies to follow (PDF):
Public Trust in A.I.
According to this first principle, A.I. could have a huge positive impact on various industries—but it also carries risks to “privacy, individual rights, autonomy, and civil liberties” (according to the White House’s document) that agencies must assess and address.
Public Participation
“Public participation, especially in those instances where A.I. uses information about individuals, will improve agency accountability and regulatory outcomes, as well as increase public trust and confidence.”
Scientific Integrity and Information Quality
Agencies must make sure that the data and tools being used in A.I. work must align with high standards of “quality, transparency, and compliance.” At the same time, government workers must take care to ensure that the data feeding various A.I. models is “of sufficient quality for the intended use.”
Risk Assessment and Management
Assessment and management of A.I. risk is apparently key in the government’s eyes: “A risk-based approach should be used to determine which risks are acceptable and which risks present the possibility of unacceptable harm, or harm that has expected costs greater than expected benefits.”
Benefits and Costs
Agencies must weigh the benefits and drawbacks of A.I. programs: “Agencies should also consider critical dependencies when evaluating AI costs and benefits, as technological factors (such as data quality) and changes in human processes associated with AI implementation may alter the nature and magnitude of the risks and benefits.”
Flexibility
This principle asks federal agencies, usually the most inflexible of organizations, to display a little adaptability when it comes to A.I.: “Rigid, design-based regulations that attempt to prescribe the technical specifications of AI applications will in most cases be impractical and ineffective, given the anticipated pace with which A.I. will evolve and the resulting need for agencies to react to new information and evidence.”
Fairness and Non-Discrimination
“Agencies should consider in a transparent manner the impacts that AI applications may have on discrimination.”
Disclosure and Transparency
Agencies should figure out how to most effectively announce when A.I. is in use, and determine if additional measures of transparency are needed once an A.I. tool is integrated into a workflow.
Safety and Security
“Agencies should promote the development of AI systems that are safe, secure, and operate as intended.” Nobody build Skynet! That would be bad!
Interagency Coordination
“A coherent and whole-of-government approach to A.I. oversight requires interagency coordination.” In other words, agencies should share data, policies, and experiences with A.I. work.
A.I.: Where Do We Go From Here?
Will the various agencies follow the White House’s principles? The federal government does not have the best reputation for moving speedily, to put it mildly, although it has more than enough funding to guarantee perpetual research and development into what it views as key technology areas. Let’s not forget that federal tax dollars fueled research into the creation of robust, fault-tolerant computer networks in the 1960s—which would eventually lead to the rise of the Internet and the World Wide Web.
Like any cutting-edge technology, A.I. and machine learning are experiencing growing pains of a sort. For example, IBM’s Watson platform—despite its early hype—has faced some challenges in attempting to more effectively diagnose and treat cancer. Uber tried to become one of the pioneers in autonomous driving, a particularly high-stakes branch of A.I.—only to slam the brakes on its efforts when a self-driving car accidentally killed a pedestrian.
In a notable blog posting, Filip Piekniewski, an A.I. researcher, once evocatively described how the artificial intelligence hype has greatly exceeded the reality, including a lack of progress in Google’s DeepMind, as well as issues with deep learning (“does not scale,” he concluded). In Google’s defense, its incremental approach to A.I. has resulted in some promising advances in both self-driving and healthcare diagnosis, but over-hype remains an issue that the A.I. industry as a whole will no doubt continue to struggle with.
A “light touch” by federal agencies, combined with federal funding (when appropriate) may help accelerate the A.I. field past some of its current issues—but will fewer regulations indeed trigger unintended consequences?