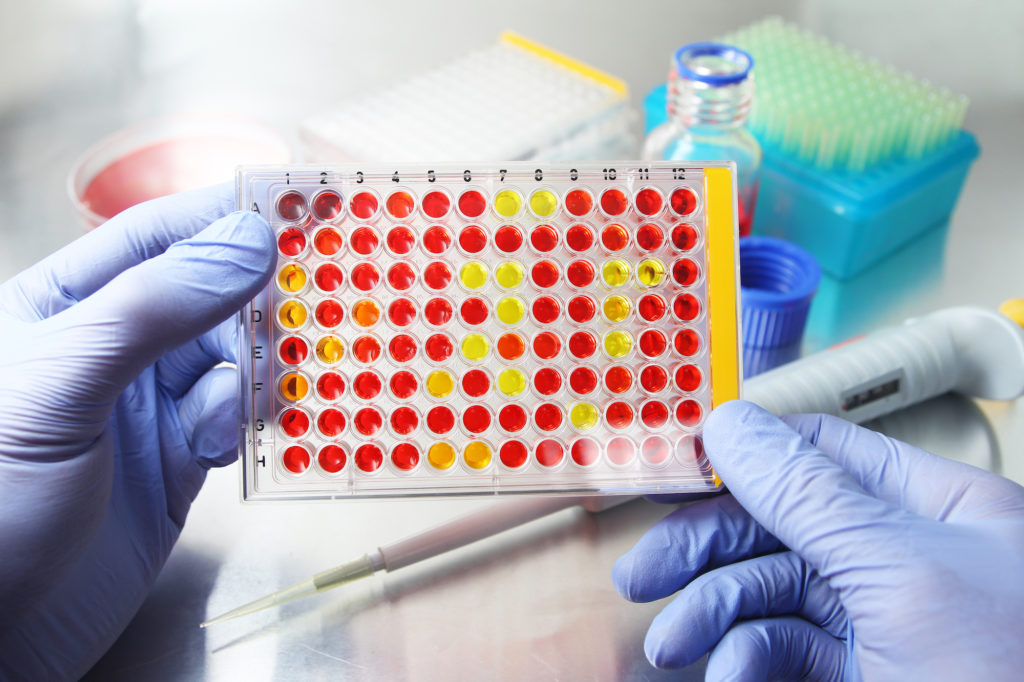
The science of data analytics is about extracting value or “information” from bits and bytes. While there is a lot of hype about Big Data and artificial intelligence (A.I.), perhaps it is more important to view these emerging technologies in terms of what specific problems they can solve for humanity.
Drugs are Too Expensive
The
U.S. pharmaceutical industry is estimated at $446 billion, representing 45 percent of the global market share. In the U.S., we pay
three times more for drugs than Britain, 6 times more for drugs than Brazil, and up to 16 times more than countries such as India. This price disparity is largely concentrated in older drugs before they reach generic level, and might be due to the fact that the U.S. allows a free-market drug economy as compared to European countries that often cap drug costs. Drug manufacturers point to high-risk research and development efforts as the cause of drug pricing. In U.S. pharmaceuticals,
R&D comprises 20 percent of total company revenues, a $58.8 billion industry in 2015. Pharmaceutical companies aim for
2-3 new molecular entities per year to justify rising R&D costs, with a overall success rate of 4.1 percent for what is, on-average, a 14-year process. But in recent years, pharmaceutical companies have had difficulty meeting these targets despite
rising R&D expenditures. Pre-1990s, each new approved drug cost $250 million; by the 2000s, costs had risen to $403 million per drug; in 2010 these costs had reached $873 million—and they continue to rise. These costs are distributed between discovery and preclinical development (33 percent), clinical development (63 percent), and submission to launch (5 percent). Additionally, costs capitalize over the average 14-year approval process, leading to a reported capitalized cost of $1.778 billion per drug in 2010. The
reasons for the high risk/reward ratio in drug development are complex but they include:
- Preclinical timelines have increased.
- Extended FDA review with the Prescription Drug User Fee Act (PDUFA).
- Lack of reliability in published data.
- Poor predictive models in preclinical R&D.
- The switch to “target-based drug discovery” adds complexity in target selection.
- Clinical trials for chronic disease are more complex.
- Outsourcing Phase 1 discovery to smaller organizations improves innovation, but results in lower probability of success due to lack of pooled knowledge.
Three disruptive innovations have the potential to dramatically reduce the cost of drug development in the United states. These are artificial intelligence programs, Big Data (and more specifically the
structuring of Big Data), and blockchain technology.
Artificial Intelligence
Artificial intelligence software has evolved. First-wave A.I. programs were simply more complex optimization programs or “knowledge engineering” software. Second-wave A.I. was comprised of statistical learning programs or “machine learning,” and achieved targeted success in pattern recognition for complex data (i.e., voice recognition, retinal scans, etc.). But third-wave AI is a major disruptor; this hypothesis generation or “contextual normalization” software has the potential to delve into Big Data, find the statistical patterns in it, then generate novel algorithms that explain why these patterns exist. Pharmaceutical companies are investing in
open innovation, and this means increased collaboration with smaller, nimbler, companies. In an ideal world, a small company would have access to the same high-quality drug-development information as a large company. A.I. software has the potential to bridge this gap and give a competitive edge to a smaller company. Additionally, third-wave A.I. can parse loose associations from previously disconnected contexts for meaning. Weak-tie networks have a high cognitive distance, and therefore a high potential for radical innovation. Ties that are strong have a lower cognitive distance and thus are lower yield. Radical innovation is about identifying weak links in the information ecosystem and finding cross-industry partners that bring a host of capabilities to open innovation.
Big Data
When people talk about Big Data, they are talking about access to large-scale digital information that is unstructured. Biological data is deep, dense, and diverse. While pharmaceuticals are traditionally approved based on proprietary and highly curated datasets from clinical trials, population drug use generates data that is much more complex. Almost a third of U.S. drugs have
side effects that are only measured years after approval with widespread population use. This phenomenon slows the approval of drugs and results in costly post-approval monitoring efforts. Now, with the advent of
electronic medical record (EMR) systems in medicine,
smart pills that can be tracked through the human gut,
DNA repositories, and
health & fitness apps which measure everything from sleep to blood pressure, we are gaining access to large scale unstructured datasets of real-time information. Before, this data had to be manually collated by humans into checkboxes, diagnosis codes, or reporting databases; now it can be automatically structured, which greatly increases the amount of data that can be analyzed for patterns.
Blockchain
Blockchain is not meant to replace centralized systems where unified organizations deliver a coordinated product. Instead, it provides a tamper-proof digital ledger whenever collaborating parties have competing interests. Originally developed for cryptocurrency, the technology represents a societal evolution. When a group of interested parties agree to record transactions in a transparent, chronological, mutually accessible format, middlemen guarantors are reduced, audibility improves, and the natural byproducts are systematically improved trust and efficiency. In many situations, government agencies, pharmaceutical companies, care providers, hospital systems, and patients are incentivized to misrepresent data; this translates into system-wide inefficiencies. U.S. pharmaceutical companies have come under fire for
price-fixing, failing to prevent
drug shortages, and
biased reporting of the results of drug trials. As an example, in 2012,
GlaxoSmithKline agreed to pay over $3 billion dollars in fraud charges for improper marketing of several pharmaceuticals. Blockchain has potential value for medical billing, pharmaceutical supply chain, and de-identified patient record transmission. However, its most immediate applicability in the life sciences is in scientific data collection for clinical trials. Using blockchain, a patient can create a “
smart contract,” where a researcher has permission for real-time data collection co-occurring with general medical treatment. With this technology, patients would be able to know their data is 100 percent secure; if the drug ever goes to market, they could receive a revenue stream for their help in contributing to the process. Early efforts to extend this to patient records have been launched, including the
Robomed platform, where participating medical providers and patients make blockchain-based smart contracts to pool access to patient records and transfer payments for medical services.
Gunjan Bhardwaj is the Founder and CEO of Innoplexus, a leader in AI, machine learning, and analytics as a service for healthcare, pharma, and the life sciences. Before founding Innoplexus, he was with the Boston Consulting Group and, prior to that, served as the leader of the global business performance think-tank of Ernst & Young and as a manager in the German practice with a solution focus on strategy and innovation.