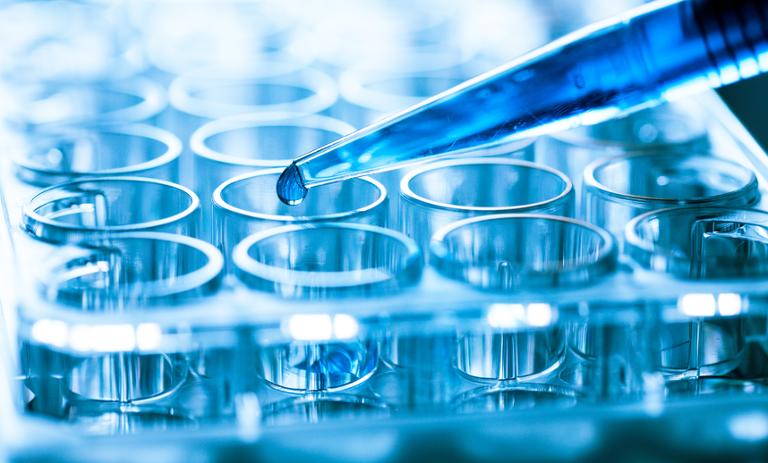
New drugs (the legal kind) undergo years of safety and effectiveness testing—which is why, according to the Association of Clinical Research Organizations (ACRO), the average drug or medical device takes 15 years and $1.2 billion to develop, test and bring to market. All that clinical testing results in a lot of data, especially in later phases when thousands of subjects are using the drug through dozens of medical facilities. “Our product is data,” said Andrew Thompson, vice president of IT architecture at Dublin-based clinical research organization Icon.
First, the Process
Drugs and medical devices go through four phases of trials. Phase 1 tests the drug on a relatively small group of healthy people to, in ACRO’s words, “determine the drug’s basic safety and pharmacological data.” Phases 2 and 3 engage steadily larger groups of patients. Together, Phases 1, 2, and 3 can take anywhere from four to seven years. Phase 4 trials are conducted after the drug has received regulatory approval, and are meant to explore additional uses, test new doses and formulations, and examine supplementary benefits the drug might offer, such as cost-effectiveness or improved quality of life for patients suffering from a certain condition. It’s easy to see why data would play a critical role in such efforts. For the most part, research organizations rely on in-house talent to handle the work of compiling, scrubbing and formatting data for presentation to the regulatory authorities who must approve the drug for market. (In the U.S., that’s the Food and Drug Administration.) Not surprisingly, as the technology behind analytics platforms has become more powerful, research organizations—which are hired by pharmaceutical companies to conduct trials of their products—have begun leveraging Big Data for more than simply tracking the results of a particular testing program.
It’s All About the Data
At Icon, Thompson says the company concerns itself with three types of data:
- Clinical data, which is collected as part of a clinical trial.
- Operational data, which is used to run the business by tracking individual projects, manage operations, measure quality control, financial results and so on.
- And data captured from paper documents, which are becoming digitized more and more.
Operational data is important for reasons that go beyond internal business reasons, Thompson said. For example, Icon stores a growing amount of historical data “that can tell us how effective it would be to start a trial in a certain place with certain investigators.” Applying analytics speeds the process by helping research organizations design trials knowing where they can find the right populations and investigators to run the trial at multiple locations. In addition, the data can “put out some pretty sophisticated visualizations” that allow the team to identify safety concerns, outliers, and track the quality of individual investigators. Such visualizations “can help you uncover issues you might not have seen before,” Thompson observed. For example, data scientists might find that every test subject whose results were submitted at a particular time from a particular facility had the same blood pressure data. “That doesn’t happen,” Thompson said. “It’s a red flag about something in that cohort and might mean we have to change the pattern of site monitoring.” In such cases, the data specialists send their findings to the people responsible for working with the medical site in question, so they can determine what’s going on and resolve any issues. That may be a big reason why most researchers rely on internal data teams to do their work. “The key is to understand the data and the context in which it’s being used,” Thompson explained. “Technology is a part of it, but the job is really about the knowledge.” With all that said, precisely how data figures into clinical research depends on the product being developed, said David Ricciardi, CEO of Proximo, a Jersey City, N.J.-based analytics consulting firm that has worked with clients in healthcare and pharmaceuticals, usually during phase 4 efforts. Once a product is on the market, he said, data scientists can correlate information in different ways to learn whether the product might have an application beyond the one for which it was originally intended. In those cases, “You’re trying to find a correlation that shows people who used a product received an unexpected benefit.” In the end, researcher focus is on the quality of their data. “We want to be complete and accurate,” Thompson said. “We maintain an ongoing assessment of outliers and have to consistently manage the data through project’s lifecycle,” which includes doing things like visiting testing sites to audit data collection and looking for patterns that raise questions.